What the Tech is media mix modeling?
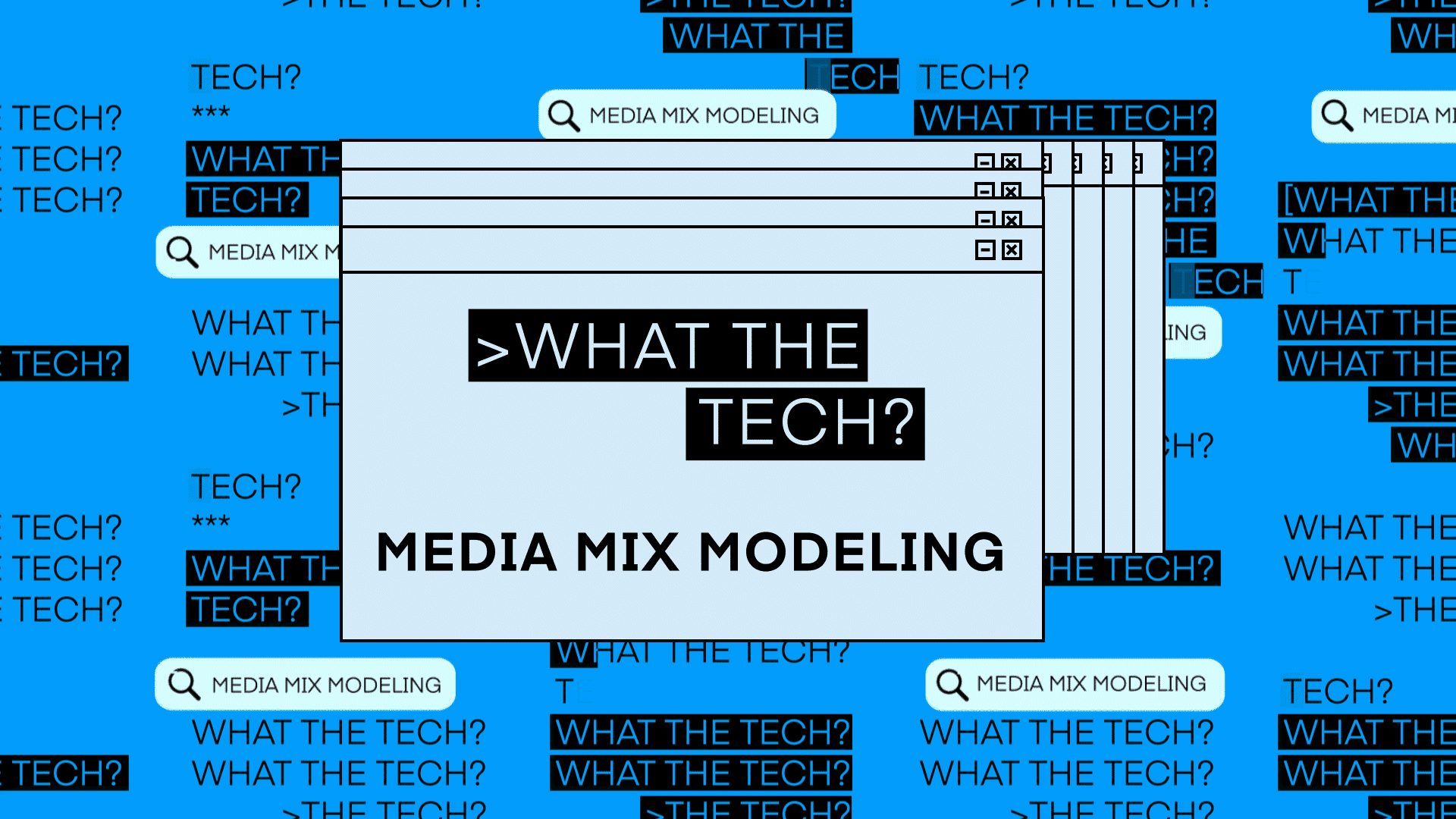
Illustration by Robyn Phelps / Getty / The Current
Taste is often cyclical. What was once cool inevitably becomes cringe, only for a new generation to reappropriate the phenomenon and make it cool again. (See: Zoomers wearing baggy jean cuts first popularized in the 1990s.)
The cyclical nature of trends can also be found in advertising measurement, where media mix modeling (MMM) has experienced an unexpected resurgence. Meta, Google and Amazon — which make up the triumvirate of digital advertising — have all embraced MMM in recent years, making it easier for marketers to use their platform data to conduct MMM analyses. It’s a surprising development considering that these so-called walled gardens are usually loath to share their proprietary data, especially when it could mean giving credit to competing platforms for driving conversions.
So why is MMM back in vogue? And why are the three major tech platforms playing nice(r) with one another?
All valid questions. We answer those, and more, below.
What is MMM?
In the simplest of terms, MMM is a way to measure the effectiveness of the various components of a multichannel advertising campaign.
Most advertising campaigns include various advertising channels — such as connected TV (CTV), social media, display and out-of-home, to name a few — in order to help maximize the reach, frequency and effectiveness of a campaign. MMM can determine how much each individual channel contributed to the overall success of the advertising campaign. Which is easier said than done.
How does MMM work?
MMM was first developed in the 1960s, when computing was first introduced to the advertising industry. Math wonks would collect reams of data about a brand’s advertising spend across various channels and compare it with the brand’s sales data in different regions. Using statistical analysis, MMM would determine how much the radio campaign affected sales versus the brand’s TV commercials versus its print advertising buys.
Conducting this analysis requires identifying different variables and establishing controls for benchmarks.
Here’s a simple example:
- A household cleaning brand runs a TV ad campaign in the American Southwest.
- The brand’s sales in the Southwest increase relative to other regions in the year following the start of the campaign.
- An MMM analysis shows that the TV campaign elicited sales, delivering a positive return on ad spend (ROAS).
Now imagine that kind of analysis conducted on all the different channels (both analog and digital) in a campaign, taking into account how much is spent on each and how each one individually contributes to sales. That’s MMM.
Why is MMM valuable?
Knowing the relative value of different advertising channels allows brands to spend their advertising budgets more wisely, cut down on ad waste and help achieve an even higher ROAS. If an MMM analysis shows that CTV is delivering a higher ROAS than, say, social media, then a brand can reallocate its marketing mix to spend more on CTV and less on social.
This holistic view of a marketing campaign is known as multi-touch attribution. For much of the history of digital media, marketers relied on last-touch attribution, which would give all the credit for a campaign’s success to the very last engagement a consumer makes before purchasing the product.
Let’s go back to the household cleaning product example. Say the brand is now running a multichannel campaign that includes podcast ads, YouTube pre-roll ads and retail media ads in Amazon. A consumer sees the brand’s pre-roll ad when viewing YouTube and hears its podcast ad while driving, but the consumer doesn’t immediately buy the product. The next week, the consumer is shopping for household cleaning products on Amazon when it comes across a sponsored listing for the brand. The consumer clicks on the sponsored listing and buys the product.
With last-touch attribution, all of the credit for that conversion would go to the Amazon sponsored ad. Surely the Amazon ad played a role in selling the product, but the consumer may have been influenced by the video and audio ads she saw and heard previously.
Multi-touch attribution models, such as MMM, aim to solve this problem by taking into account all aspects of an ad campaign and calculating the incremental value of each aspect of the ad campaign (also known as incrementality).
Why not always use MMM, then?
Because it’s typically inefficient. MMM often took years of data collecting and number crunching to arrive at an accurate analysis, and that’s a lifetime by digital media standards.
But the vast troves of data afforded by digital media have made conducting MMM analysis quicker and easier. Now brands can combine their first-party data with third-party measurement and purchase data to more precisely identify audiences that have been exposed to their ads and whether those audiences purchased their brand. Some ad tech startups claim they can now execute an MMM analysis with more than 90% accuracy in less than an hour.
Why are MMMs popular again?
Partially because technology has made MMM analysis easier (see above), but also because privacy concerns have made other forms of measurement less viable.
Meta not-so-coincidentally started developing its MMM tool around the same time Apple made it easier for iPhone owners to opt out of cross-app tracking. That tracking was vital for Meta to trace whether an ad on one of its social platforms resulted in a purchase in a different app. Amazon and Google, the two other major advertising platforms infamous for not sharing data, have also opened themselves up to MMM analysis.
It’s possible the big three have embraced MMM because they believe demonstrating the value of online advertising, even channels they don’t own, is beneficial for the entire industry, their companies included. MMM might show that, in some cases, display advertising outperforms Instagram, but it appears Meta is fine with that so long as MMM shows that digital media, in general, is an effective advertising vehicle. It could be that the big three tech platforms believe that most MMM analyses will show their platforms deliver exceptional ROAS for marketers, which is why they’re willing to play nice with MMM vendors.
The rise of MMMs is also a result of the larger issue around measurement in digital advertising. The aforementioned major players are notorious for not allowing independent, third-party measurement systems to evaluate their platforms, a situation that critics often deride as these companies “grading their own homework.” MMMs offer, in theory, a neutral way to measure not only the big three tech platforms, but any and every advertising channel available.
Subscribe to The Current
Get the latest in modern marketing straight to your inbox weekly.