Crafting the perfect blend of data
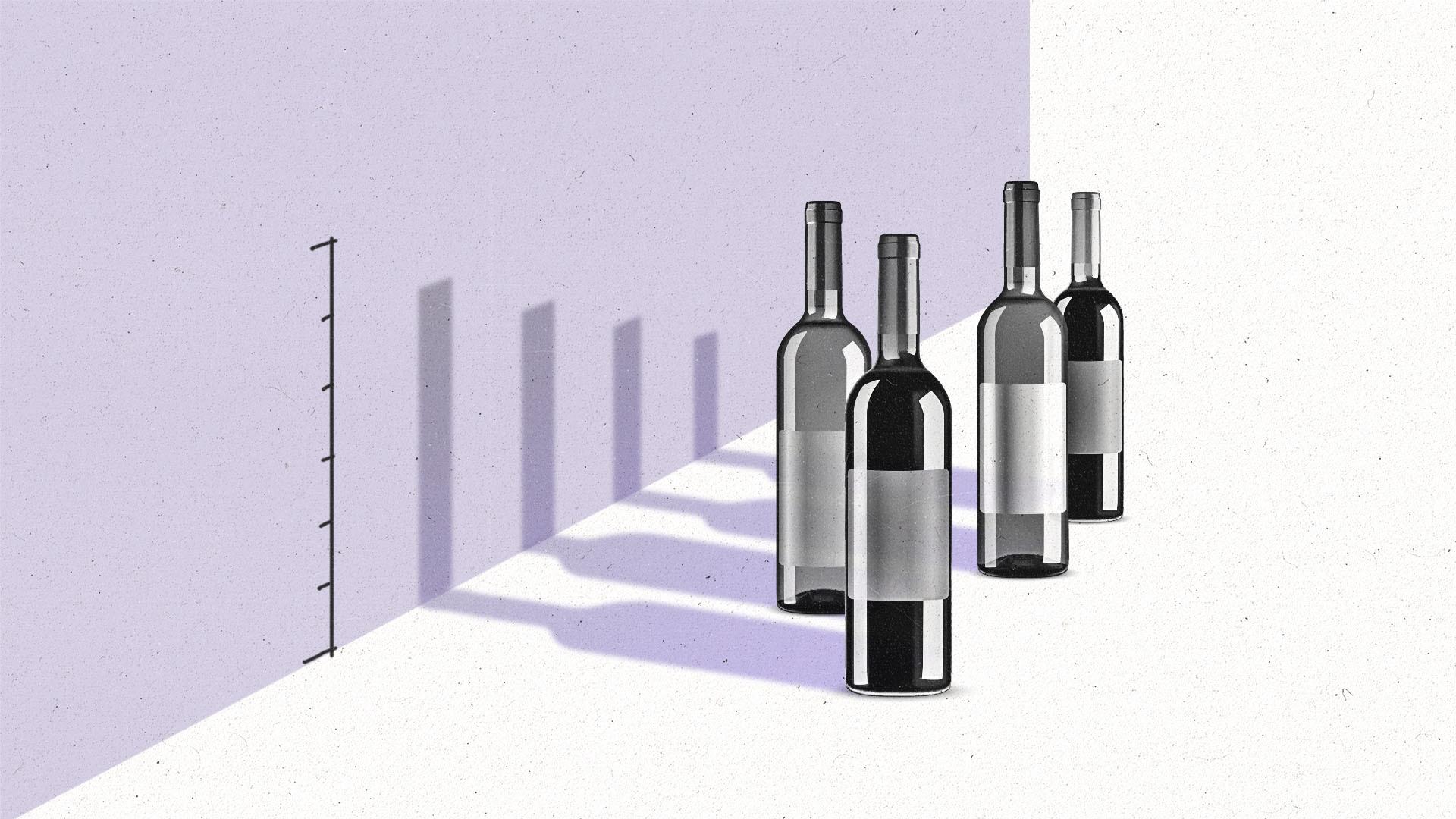
Illustration by Esther Park / Shutterstock / The Current
There is nothing like wine tasting at a vineyard, gazing into the fields while a sommelier guides you through an exclusive portfolio of wine. Your host points to a particular hillside, describing how the climate, soil, and extra care of those vines have developed luscious tasting notes. While delving into a personal story about the winemaker, they bring out a signature bottle of single-varietal, single-lot vintner’s reserve. Before even tasting it, you are hooked.
So, what makes a good wine (beyond a great story)? A balance of sweetness, acidity, tannin, alcohol, and body. Many winemakers achieve this through the art of blending, whether mixing various lots of the same varietal or blending different varietals, for example, to create a classic like a red Bordeaux.
In advertising, the data we use to improve targeting effectiveness works in much the same way. Sometimes, single-sourced data with a narrow but distinct profile may be most appropriate. But more often, blended data produces greater balance, scale, and affordability when looking to engage the broadest pool of customers and likely prospects.
However, like producing a fine wine, you can’t just throw a bunch of data into a vat and hope it comes out singing. You need to procure the right raw ingredients and have an expert team that can take their knowledge from years of study, practice, and testing to produce something you are proud to serve.
Sourcing the fruit and prioritizing quality
While picking up a bottle of wine from Italy, you may notice the label is riddled with extra words like “denominazione di origine controllata e garantita,” or “designation of origin and guaranteed.” Countries like Italy and France have strict regulations to safeguard the quality and authenticity of their wines. This is not unlike data. While it may seem that data grows on trees these days, you should only use data that has been appropriately sourced, permissioned, and follows all applicable laws and regulations.
You want to choose providers that have carefully procured high-quality data signals that can be tied back to actual consumers.
Then, blending these data sets together — with the appropriate permissions and controls to do so, gives you the data stability and ability to determine what “grapes” should be kept for a finished product and what should be left on the vine.
Achieving balance
You can begin targeting now that you’ve sourced quality data providing various attributes, such as household income. But what does household income really do for you? Does it tell you what consumers can afford, if they are in-market for a new car or shop at Whole Foods? Like wine from a single lot, it really just hits one note.
Balance comes from combining various sets of complementary data to provide more depth. This can be derived from different types of attributes, or it can come from the same kind of signal. For example, instead of using purchase insights from a single retailer, you can combine data from across hundreds of retailers, providing more complete behavioral profiles.
Maximizing yield
You can’t make one barrel of wine and expect to recoup the cost of managing an entire winery; you need these grapes to scale. As we alluded to before, data sets need to be large enough to be actionable and one of the ways we do this is through modeling. However, not all modeled audiences are created equal — you must choose a partner with access to data across various signals.
For example, if you’d like to scale back-to-school buyers, you’ll need seed data from a third party or your own first-party consumers. Next, your modeling partner should tap in to these buyers’ ID signals — like income, grocery purchases, hobbies, TV viewership, and more — to define the seed audience. Next, the modeling system will look across all data points to determine how the buyers in the seed are different from those who are not, building an equation to segment out households who are most likely to purchase back-to-school items.
Conducting taste tests
Now that your audience is built, how do you know it works? Winemakers conduct quality tests; this includes tasting before it goes into the barrel, as it ages, and even opening bottles after time have passed to determine if the wine has developed appropriately for the market.
The same rigor should go into data product solutions. In the digital world, this taste testing can be done through “holdout” testing. For example, setting aside purchase data from retailers and comparing them to your back-to-school modeled audience to determine how likely the model is to find those buyers.
Filling your wine cellar
It would be unreasonable to suggest you fill your cellar with just one wine or choose even a single winery. There are just too many quality producers out there, and each has their own specialties.
But it is wise to focus on a few as the core of your collection. The workhorses. Then, when it’s time to round out those shelves, choose a quality purveyor that does a lot of hard work curating from the many thousands of options available to give you just the right recommendations that will keep you coming back for more.
This op-ed represents the views and opinions of the author and not of The Current, a division of The Trade Desk, or The Trade Desk. The appearance of the op-ed on The Current does not constitute an endorsement by The Current or The Trade Desk.
Subscribe to The Current
Subscribe to The Current newsletter